As large-scale datasets increasingly influence critical decision-making processes in healthcare, policy, and beyond, overcoming selection bias remains a monumental challenge. Factored, in collaboration with Carnegie Mellon University, has pioneered a novel statistical framework to tackle this issue, detailed in the paper Statistical Inference Under Constrained Selection Bias.
This framework integrates user-specified constraints derived from domain expertise—such as aggregate population data—to improve the reliability of statistical inferences made on biased datasets. By introducing computationally efficient methods to manage partial information and selection bias, the work ensures scalability and applicability across domains, from public health to algorithmic fairness. Co-authored by Factored’s Carlos Miguel Patiño, the research exemplifies technical rigor and innovative problem-solving.
The team’s methodology shines in its ability to adapt real-world constraints into a mathematically rigorous optimization framework. This innovation allows organizations to make informed decisions even when data sources are inherently limited or skewed by factors like socioeconomic disparities or selective sampling.
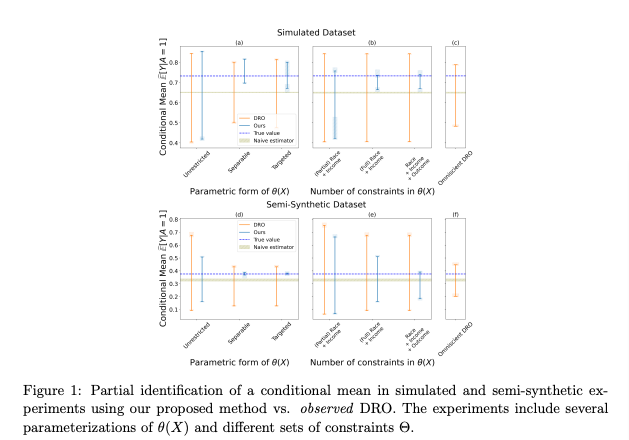
Empirical Validation: From Synthetic Data to Real-World Applications
The framework was rigorously tested on synthetic datasets and semi-synthetic data, such as the Folkstables dataset derived from U.S. Census data. Factored’s role in scaling the framework to real-world applications was pivotal, ensuring it could adapt complex domain constraints into mathematically robust optimization solutions.
One of the most impactful applications of this research involved a real-world case study on disparities in COVID-19 hospitalizations. Using over five million anonymized medical claims, the method revealed elevated hospitalization risks for Black, Asian, and Hispanic patients compared to white patients, while highlighting the challenges posed by selection bias in healthcare data.
To see the full paper click here.