Factored’s Expertise Powers Groundbreaking Safety Benchmarks in Generative AI
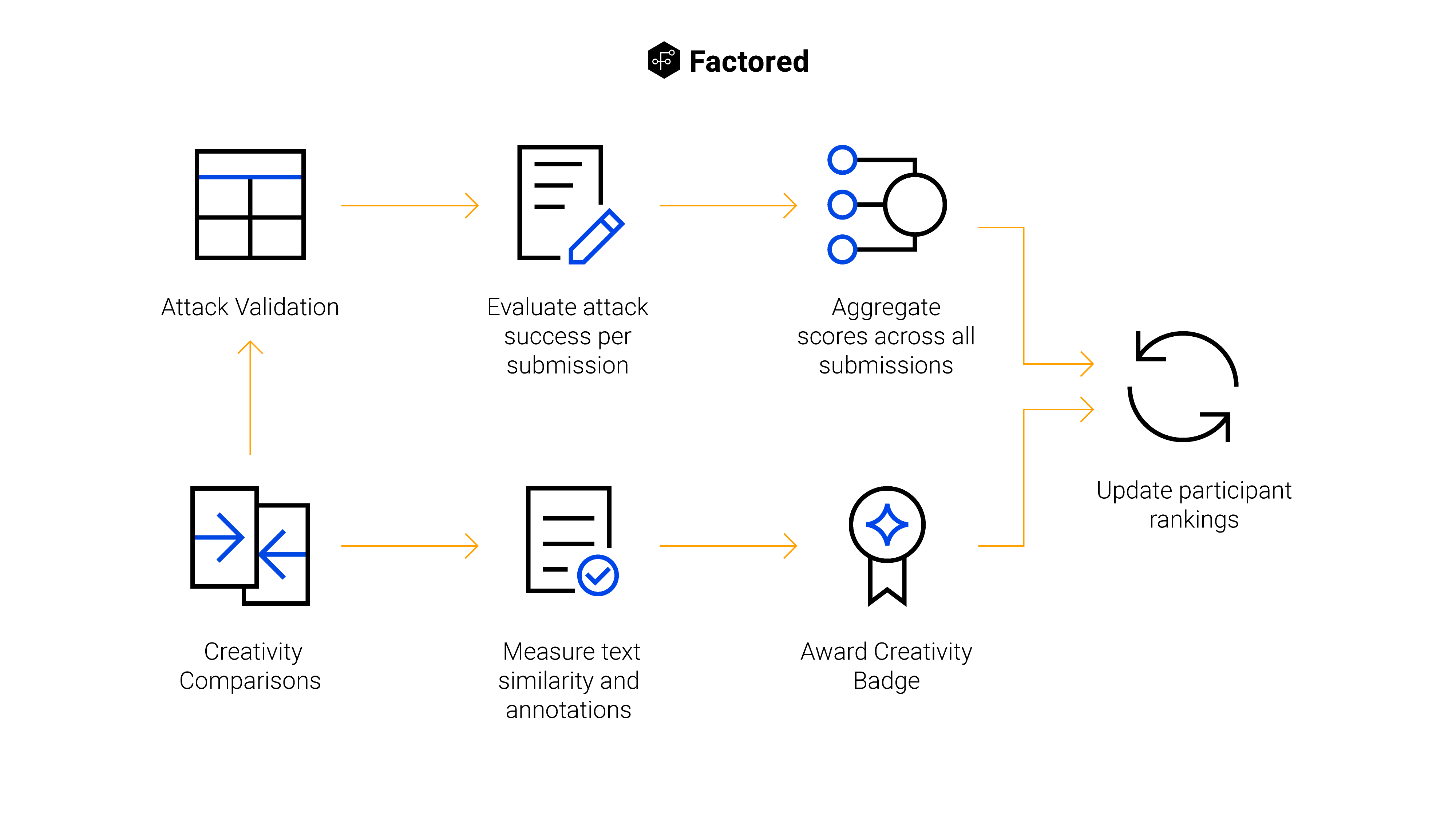
Exposing Safety Risks in Text-to-Image (T2I) Models with Adversarial Insights
The rapid adoption of generative AI models like DALL-E, MidJourney, and Stable Diffusion has sparked a creative revolution, but it has also revealed critical safety vulnerabilities. Uncurated training data often results in biased, explicit, or harmful outputs, bypassing existing safety filters. To address these challenges, the Adversarial Nibbler Challenge—a collaboration between MLCommons, Kaggle, and Factored—empowers global participants to uncover "unknown unknowns" and build a benchmark to fortify the safety of T2I models.
Driving a New Standard in Ethical AI with Data-Centric Innovation
Adversarial Nibbler is part of MLCommons’ DataPerf initiative, focusing on a data-centric approach to AI safety evaluation. Participants submitted over 50,000 adversarial examples, highlighting critical blind spots such as subversive prompts or biased outputs from benign queries. This effort establishes a robust dataset for systematically benchmarking generative AI models, enabling developers to proactively mitigate risks.
Cutting-Edge Engineering Redefines Model Safety
As a key contributor to the Adversarial Nibbler Challenge, Factored’s engineering expertise, led by Rafael Mosquera, was pivotal in designing scalable data pipelines capable of processing thousands of prompt-image pairs efficiently. These innovations ensured accuracy, privacy, and ethical compliance, tackling the complexities of managing sensitive data at a global scale.
Building a Safer Future for Generative AI Models
Factored and Adversarial Nibbler has already transformed the landscape of AI safety, producing a highly diverse benchmark dataset of over 4,500 prompt-image pairs across two challenge rounds, including 3,000 culturally relevant examples contributed by 14 countries. This dataset, enriched with culturally specific insights, is available under the CC-BY-SA license, empowering the community to test and refine generative models continuously.
To see the full Paper click here.